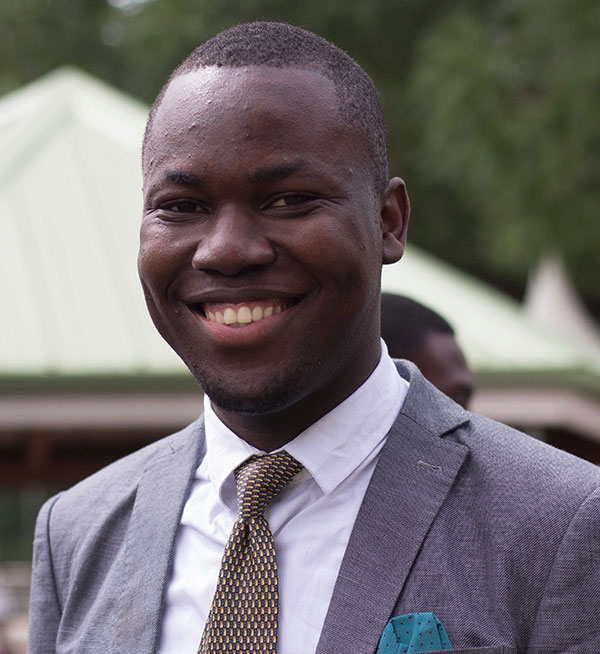
Program
MPhil Scientific Computing & Industrial ModelingGraduating Class of
2016Research Interests
Data Science, Statistical Data Analysis and Visualization, Machine Learning, Human Computer Interaction.Dissertation(s)
Affiliate Institution
Kwame Nkrumah University of Science and TechnologyDegree Obtained
BSc MathematicsEmail
reklu@nims.edu.gh
Profile
Rudolph Elikem Klu was part of the 2013 graduating cohort of the College of Science of the Kwame Nkrumah University of Science and Technology, Ghana. He graduated with a BSc Mathematics degree (First Class). His undergraduate dissertation was on "Prognosis of Malignant Melanoma Disease by Image Analysis". He went on to serve as a Teaching Assistant in the Department of Mathematics, for the duration of his mandatory National Service. He also obtained in July 2017, an MPhil degree in 'Scientific Computing and Industrial Modelling', being a part of the first graduating cohort of the 'Scientific Computing and Industrial Modelling' programme with the National Institute for Mathematical Sciences, Ghana.
His research interests include Data Science, High Performance Computing, Numerical Analysis, Differential Equations, Statistical Data Analysis and Visualization, Artificial Intelligence, Machine Learning, and Human Computer Interaction. His postgraduate dissertation was on "Finger-spelling Gesture Recognition Using Principal Components Analysis". This study was aimed at advancing the use of computing resources to recognize hand spelling gestures to aid the deaf/mute communicate with non-signers.
Rudolph is an excellent team player with great communication skills. He is also very motivated, and perfectly capable of undertaking independent work. His ultimate objective is to apply the knowledge he has obtained in computing and mathematics thus far to the fields of Data Science, Machine Learning and Human Computer Interaction, geared towards Business Innovation and Corporate advancement. His other passions include music, playing the guitar, teaching and mentoring, and graphics designing.
Research Summary
Sign language is one of the most natural and raw forms of language and communication. which could be dated back to as early as the advent of the human civilization, when the first theories of sign languages appeared in history. This thesis presents an approach to recognizing hand spelling gestures to aid the deaf/mute communicate with non-signers.
Images were captured using a computer camera. Skin/hand areas were segmented out of the images using color, rotated onto their principal axis by the method of moments and transformed into a PCA feature space for gesture feature identification and characterization.
Data was trained in this system and subsequently test data was classified using a single space Euclidean classifier and a single space Mahalanobis classifier which utilized the Euclidean and Mahalanobis distances respectively. The two classifiers are compared for their accuracy and efficiency. The results of the work indicated that the single space Mahalanobis classifier performed better than the single space Euclidean classifier especially as the number of principal components increased. The number of principal components selected also greatly affected the accuracy of classification, with more principal components introducing noise in the images.