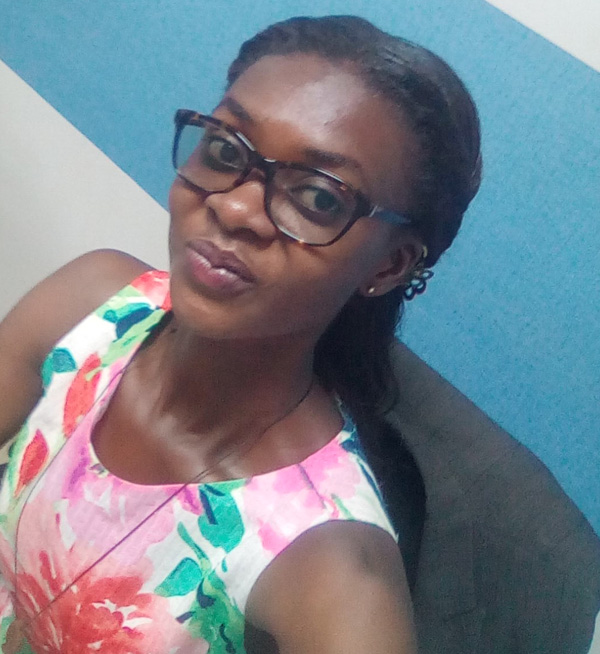
Program
MPhil Scientific Computing & Industrial ModelingGraduating Class of
2018Research Interests
Differential Equations, Forensics and Intelligent AnalysisDissertation(s)
Affiliate Institution
Kwame Nkrumah University of Science and TechnologyDegree Obtained
BSc. MathematicsEmail
mgamankwah@nims.edu.gh
Profile
Mercy Gyamea Amankwah holds a BSc Mathematics degree from the Kwame Nkrumah University of Science and Technology, Ghana, obtained in July 2015. Subsequently, she was a Teaching Assistant with the Department of Mathematics of the Kwame Nkrumah University of Science and Technology, Ghana, for her one year mandatory National Service.
Mercy is currently a postgraduate research student with the National Institute for Mathematical Sciences, Ghana, in the 'Scientific Computing and Industrial Modelling' programme. Her research interests lie mainly in the area of Differential Equations, and she aspires to delve into Forensics and Intelligence Analysis.
Research Summary
In crime investigation and other disciplines, details in images are of much importance. However, images are usually degraded by blur and noise or either of the two. Given that several deblurring and denoising algorithms have been developed and some more of these are yet to be born. This work has reviewed some of these deblurring algorithms in detail, namely: inverse filtering, Wiener filtering and Constrained Least Squares filtering. The performance of these methods has been compared by first subjecting them to a motion blur (length=9, angle=5˝ ) at a standard blur length and noise level. This revealed an abysmal performance of the Inverse filter in the presence of noise. Wiener filtering and Constrained Least Squares filtering were further examined by varying the blur lengths. On choosing regularization parameters that have values less than 0.02, Constrained Least Squares filtering produced smaller Mean Squared Errors than the Wiener filtering. A mean squared error of 7.0001e ´03 was recorded for a regularization parameter of value 0.02. Furthermore, this work investigates the regularization parameter under the Constrained Least Squares filtering by applying the method to the same image subjected to a wide range of blur levels. Last but not least, image denoising is performed on the deblurred image produced by Constrained Least Squares method with a regularization parameter of value 0.001 using the Average filter and Gaussian filter.