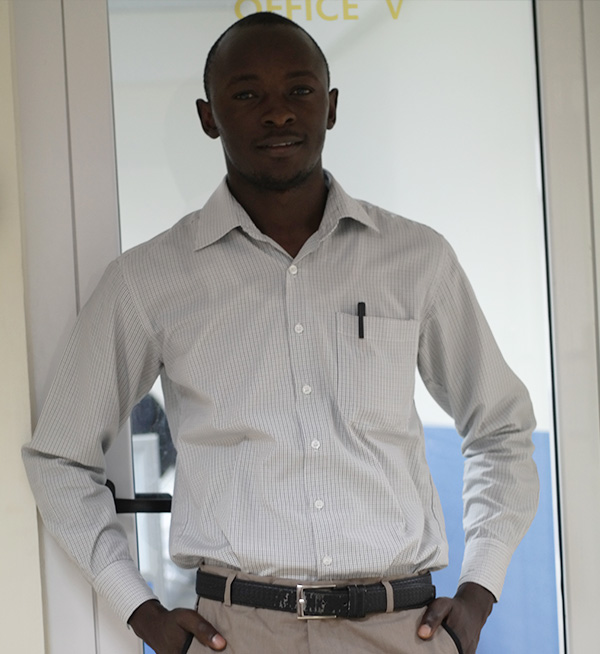
Program
MPhil Scientific Computing & Industrial ModelingGraduating Class of
2018Research Interests
Machine LearningDissertation(s)
Affiliate Institution
Jomo Kenyatta UniversityDegree Obtained
BSc Mathematics and Computer ScienceEmail
hkmsatsi@nimsedu.gh
Profile
Haward Ketoyo Msatsi attended the Jomo Kenyatta University in Kenya, where he graduated with a Bachelors Degree in Mathematics and Computer Science. He went on to attend the African Institute for Mathematical Sciences, Senegal, for postgraduate studies. He is currently enrolled in the 'Scientific Computing and Industrial Modelling' postgraduate programme of the National Institute for Mathematical Sciences, Ghana. His research interests are Machine Learning and Pattern Recognition.
Research Summary
The primary site of a given cancer and its histopathology are two major factors that predict the clinical behaviour of a human cancer. However due to metastasis, primary and secondary sites can not be distinguished in some instances even after a careful radiological examination. It becomes a great challenge to the effectiveness of the cancer treatment method used since some treatment methods like surgery are applied depending on the nature of the primary site of the cancer. If the primary site of a given cancer is identified correctly, suitable treatment for the cancer in question may be applied making the treatment method more effective. There has also been an enormous increase in the amount of sequenced gene data due to the development in sequencing technologies. However application of simple linear classifiers on the numerous data available is a major obstacle in improving classification performance. To address these challenges, a cancer primary site classifier was developed by applying the Artificial Neural Network (ANN) algorithm on somatic mutation data from the Catalogue of Somatic Mutations in Cancer (COSMIC). The classifier was able to classify cancer sites that are primarily metastasized by breast cancer with an accuracy of 77.03\%. Further more, a combination of genes and mutation descriptions was found to be the best feature combination for classification of cancer primary sites.