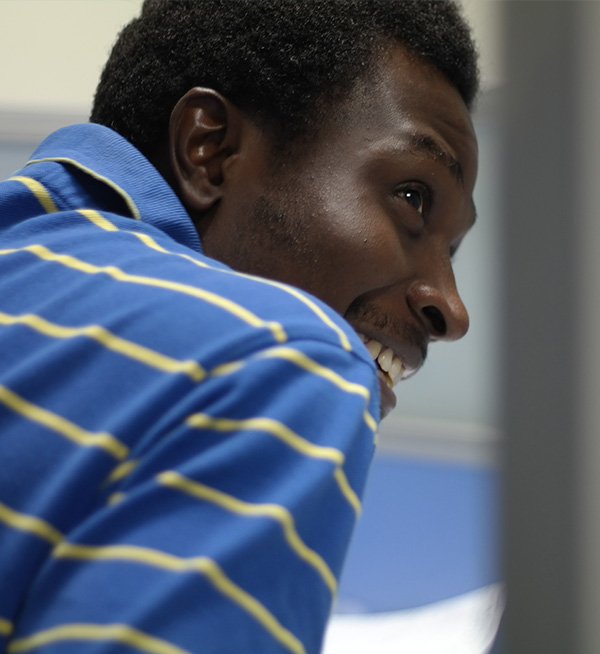
Program
MPhil Scientific Computing & Industrial ModelingGraduating Class of
2018Research Interests
Artificial IntelligenceDissertation(s)
Affiliate Institution
Kwame Nkrumah University of Science and TechnologyDegree Obtained
BSc. MathematicsEmail
eboturkson@nims.edu.gh
Profile
Michael Emmanuel Ebo Turkson graduated from the Kwame Nkrumah University of Science and Technology, Ghana, in 2015, with a BSc Mathematics degree. Outside of the regular school curriculum, he acquired technical expertise in software development, algorithm design and performance optimization from several internships and work experience. While also playing the role of Firefox Student Ambassador at his University. During his mandatory year of National Service, he worked as a Teaching Assistant with the Department of Mathematics at KNUST. He recently completed an MPhil in Scientific Computing and Industrial Modelling facilitated by The National Institute for Mathematical Sciences, Ghana. For years Ebo has been interested in Numerical Methods for computational mathematics but more recently this has changed towards Computer Vision and all things Machine Learning.
As a side project with some friends, he is digitizing Ghanaian languages for automatic language translation, beginning with Asante Twi. He hopes to one day be able to build a framework for simple digitization of under-resource languages.
Michael dreams of the day when there exists a strong bond between academia and industry in Africa and is constantly on the lookout for collaborators with similar vision. He looks forward to helping build a thriving Machine Learning community in Ghana.
Research Summary
In this work, the efficiency of convolutional neural networks is considered in selecting a feature extraction method for the automatic analysis of the surveillance footage.
A combination of a MobileNet V2 Convolutional Neural Network and a Single Shot Detector is combined to perform object detection in surveillance video. The architecture is trained and evaluated on the Microsoft Common Objects in Context dataset. It is shown that the combined architecture yields the expected results in efficiency and a mean Average Precision of 22.