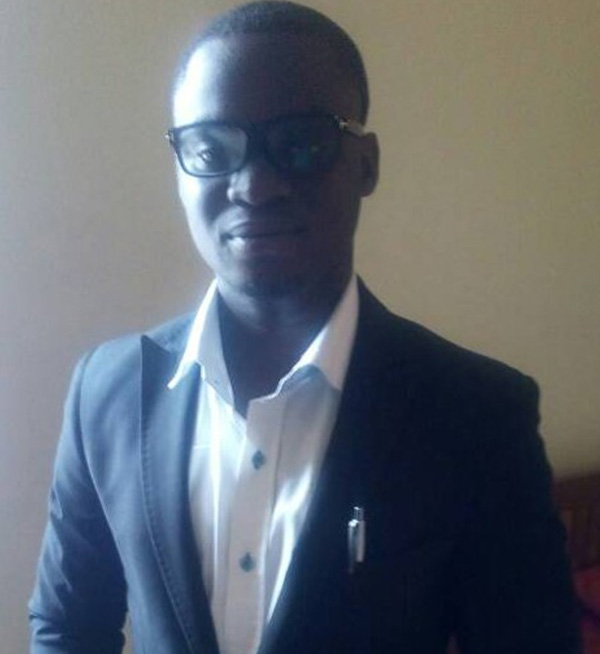
Program
MPhil Scientific Computing & Industrial ModelingGraduating Class of
2018Research Interests
Data ScienceDissertation(s)
Affiliate Institution
University for Development StudiesDegree Obtained
BSc. Financial MathematicsEmail
fkowusu@nims.edu.gh
Profile
Owusu Kofi Frank is an alumnus of the University for Development Studies, where he graduated with a BSc Financial Mathematics degree in 2015. His undergraduate thesis was in the area of Time Series Analysis. He subsequently served as a Teaching Assistant at the Department of Mathematics, of the University for Development Studies, for his one year mandatory National Service. The courses he assisted with included Set Theory, Investment Analysis and Portfolio Management, Mathematical Modelling and Financial Derivatives.
Frank is currently enrolled in the 'Scientific Computing and Industrial Modelling' postgraduate programme of the National Institute for Mathematical Sciences, Ghana. His current field of interest is Data Science.
Research Summary
Electricity has become a major part human life, especially in our part of the world. It is one of the most used energy across the world. Due to the fast changing world, the demand for electricity keeps on increasing from time to time yet there isn't any efficient way of storing this energy for future use. So operators are very cautious about the amount to release and also to meet the demand of their consumers. For this reason, load forecasting has become a main integrated section in energy management and production. This research seeks to look at Short-Term Load Forecasting. The electricity transmission company, Ghana Grid Company Limited (GRIDCo) was considered for this study. The aim was to forecast the peak demand and total energy generated or electricity demand.So the Seemingly Unrelated Time Series Equations Model which models the level or state and trend in the system was used for the study. A Markov Chain Monte Carlo (MCMC) method, Gibbs Sampler, together with the Kalman Filter and Kalman Smoother, the Forward Filtering Backward Sampling with Gibbs Sampler Algorithm was applied to the model to simulate for the variances also to predict the peak demand the next day's peak and electricity demand. The one-step-ahead forecast showed a Mean Absolute Percentage error (MAPE) of 3.696% error in the peak demand forecast and a 4.235% error in the electricity demand forecast. The forecast for the next day was about 2187MW and 44090MW for the peak and electricity demands respectively.